Step 7: Connecting Deep Lake Datasets to ML Frameworks
Connecting Deep Lake Datasets to machine learning frameworks such as PyTorch and TensorFlow.
How to use Deeplake with PyTorch or TensorFlow in Python
Deep Lake Datasets can be connected to popular ML frameworks such as PyTorch and TensorFlow, so you can train models while streaming data from the cloud without bottlenecking the training process!
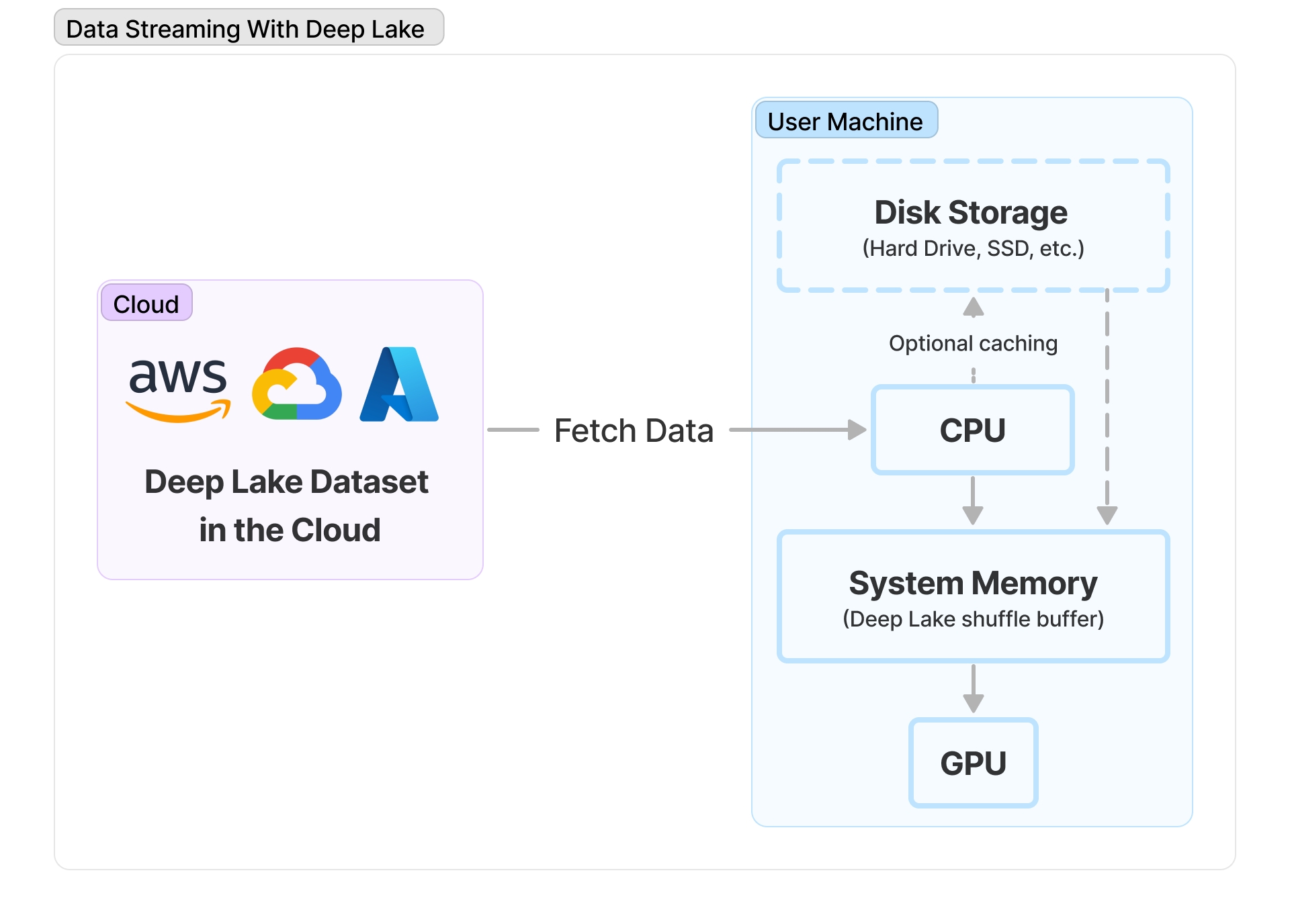
Training models with PyTorch
There are two syntaxes that can be used to train models in Pytorch using Deep Lake datasets:
Deep Lake Data Loaders are highly-optimized and unlock the fastest streaming and shuffling using Deep Lake's internal shuffling method. However, they do not support custom sampling or fully-random shuffling that is possible using PyTorch datasets + data loaders.
Pytorch Datasets + PyTorch Data Loaders enable all the customizability supported by PyTorch. However, they have highly sub-optimal streaming using Deep Lake datasets and may result in 5X+ slower performance compared to using Deep Lake data loaders.
1. Deep Lake Data Loaders for PyTorch
Best option for fast streaming!
The fastest streaming of data to GPUs using PyTorch is achieved using Deep Lake's built-in PyTorch dataloader ds.pytorch()
. If your model training is highly sensitive to the randomization of the input data, please pre-shuffle the data, or explore our writeup onShuffling in dataloaders.
import deeplake
from torchvision import datasets, transforms, models
ds = deeplake.load('hub://activeloop/cifar100-train') # Deep Lake Dataset
Transform syntax #1 - For independent transforms per tensor
The transform
parameter in ds.pytorch()
is a dictionary where the key
is the tensor name and the value
is the transformation function for that tensor. If a tensor does not need to be returned, the tensor should be omitted from the keys. If no transformation is necessary on a tensor, the transformation function is set as None
.
tform = transforms.Compose([
transforms.ToPILImage(), # Must convert to PIL image for subsequent operations to run
transforms.RandomRotation(20), # Image augmentation
transforms.ToTensor(), # Must convert to pytorch tensor for subsequent operations to run
transforms.Normalize([0.5, 0.5, 0.5], [0.5, 0.5, 0.5]),
])
#PyTorch Dataloader
dataloader= ds.pytorch(batch_size = 16, num_workers = 2,
transform = {'images': tform, 'labels': None}, shuffle = True)
Transform syntax #2 - For complex or dependent transforms per tensor
Sometimes a single transformation function might need to be applied to all tensors, or tensors need to be combined in a transform. In this case, you can use the syntax below to perform the exact same transform as above:
def transform(sample_in):
return {'images': tform(sample_in['images']), 'labels': sample_in['labels']}
#PyTorch Dataloader
dataloader= ds.pytorch(batch_size = 16, num_workers = 2,
transform = transform,
tensors = ['images', 'labels'],
shuffle = True)
2. PyTorch Datasets + PyTorch Data Loaders using Deep Lake
Best option for full customizability.
Deep Lake datasets can be integrated in the PyTorch Dataset class by passing the ds
object to the PyTorch Dataset's constructor and pulling data in the __getitem__
method using self.ds.image[ids].numpy()
:
from torch.utils.data import DataLoader, Dataset
class ClassificationDataset(Dataset):
def __init__(self, ds, transform = None):
self.ds = ds
self.transform = transform
def __len__(self):
return len(self.ds)
def __getitem__(self, idx):
image = self.ds.images[idx].numpy()
label = self.ds.labels[idx].numpy(fetch_chunks = True).astype(np.int32)
if self.transform is not None:
image = self.transform(image)
sample = {"images": image, "labels": label}
return sample
The PyTorch dataset + data loader is instantiated using the built-in PyTorch functions:
cifar100_pytorch = ClassificationDataset(ds_train, transform = tform)
dataloader_pytroch = DataLoader(dataset_pt, batch_size = 16, num_workers = 2, shuffle = True)
Iteration and Training
You can iterate through both data loaders above using the exact same syntax. Loading the first batch of data using the Deep Lake data loader may take up to 30 seconds because the shuffle buffer is filled before any data is returned.
for data in dataloader:
print(data)
break
# Training Loop
for data in dataloader_pytorch:
print(data)
break
# Training Loop
For more information on training, check out the tutorial on Training an Image Classification Model in PyTorch
Training models with TensorFlow
Deep Lake Datasets can be converted to TensorFlow Datasets using ds.tensorflow()
. Downstream, functions from the tf.Data
API such as map, shuffle, etc. can be applied to process the data before training.
ds # Deep Lake Dataset object, to be used for training
ds_tf = ds.tensorflow() # A TensorFlow Dataset
Was this helpful?